1. Model representation
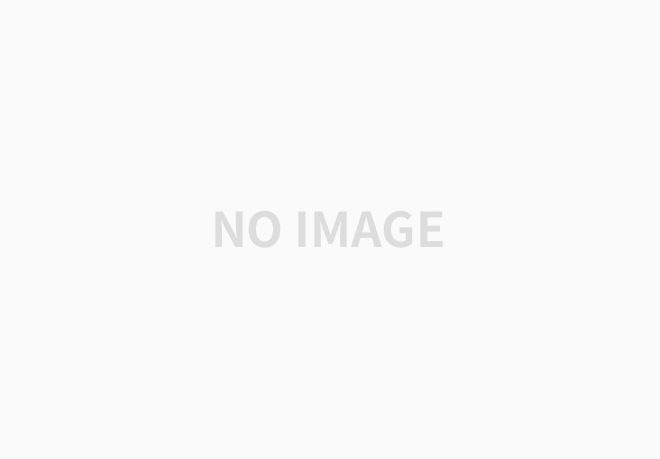
Regression의 목표는 주어진 data(파란색)를 분석해서 새로운 Input에 대해 알맞은 결과를 예측하는 것이다. 그중 Linear regression은 결과를 연속적으로 예측할 수 있도록 빨간 선과 같은 알맞은 함수 모델을 찾는 것이 목표이다. 모델을 찾는 과정은 다음과 같다.
1. Function Hypothesis :
hθ(x)=θ0+θ1x1+θ2x2+⋯
2. Cost Function :
J(θ0,θ1)=12m∑mi=1(hθ(x(i))−y(i))2
3. Find parameters that minimize the cost fuction -> Gradient Descent
4. Use hypothesis as a model
2. Gradient Descent
어떤 함수가 convex일 때, minimum 찾는 것을 가능하게 해주는 방법이다. 간단하게 설명하면, paprameter 값을 조정하면서 함수의 기울기가 0으로 변하지 않는 곳을 찾는 것이다. 따라서 함수는 convex여야만 한다.
parameter 조정은 다음과 같은 알고리즘을 parameter가 수렴할 때까지 반복하는 것으로 가능하다.
θj:=θj−α[ddθjJ(θ0,θ1,⋯)]
(θj: 임의의 값(0, 1 등)부터 시작, α: learning rate(>0))
개념을 잡기 위해 다음과 같은 convex 함수에 Gradient Descent를 적용해 최솟값을 가지게하는 parameter 값을 얻는 과정을 간단하게 확인해보자.
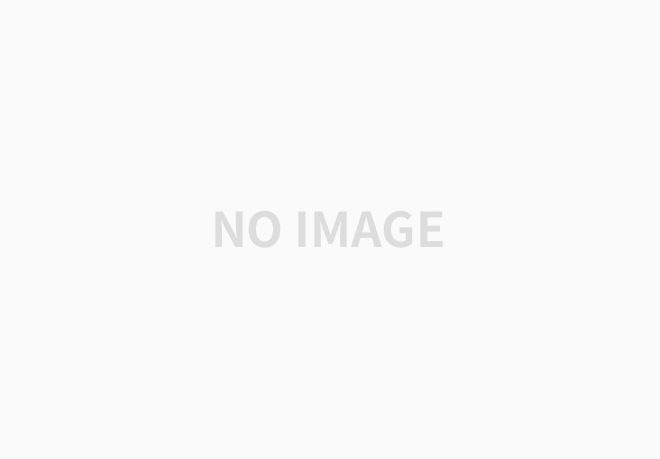
우선 θ1,θ2,⋯에 아무 값이나 넣어서 Gradient Descent를 수행한다. θ1=−1이라고 하자.
θ1:=−1−αdJdθ1
θ1:=−0.5−αdJdθ1
θ1:=−0.3−αdJdθ1
θ1:=−0.1−αdJdθ1
⋯
−αdJdθ1>0 이므로 new θ1> old θ1이 된다. 이 움직임은 그래프 위에서 다음과 같다.
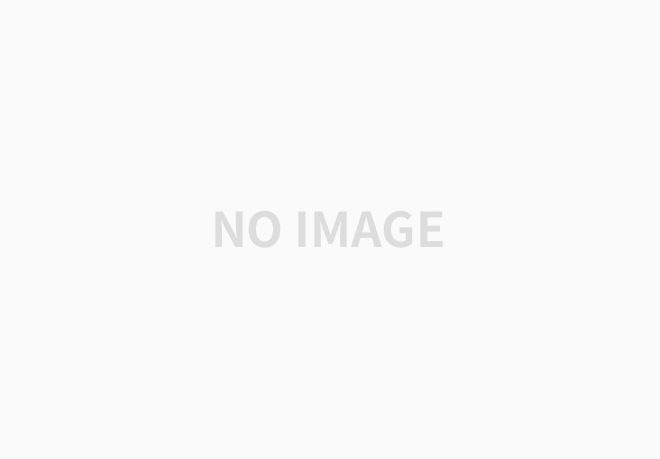
움직임의 정도는 α에 의해 결정된다. α가 크다면 큰 폭으로 parameter 값이 조정되며 α가 작으면 작은 폭으로 값이 조정된다. 일반적으로는 초반에는 α를 큰 값으로 설정하고 이후에 α 값을 줄여가면서 Gradient Descent를 사용한다.
3. Gradient Descent Algorithm
위 과정을 정리하면 다음과 같이 나타난다.
Hypothesis: hθ(x)=θTx=θ0x0+θ1x1+⋯+θnxn
Parameters: θ0,θ1,⋯,θn
Cost function: J(θ0,θ1)=12m∑mi=1(hθ(x(i))−y(i))2
Gradient descent:
repeat {
θj:=θj−α1m∑mi=1[hθ(x(i))−y(i)]∗x(i)j
}
4. Feature Scaling
hθ(x)=θTx에서 0<x1<5000,0<x2<10처럼 범위가 주어지면, parameter θ보다 x 값의 영향이 커지고 이로 인해 parameter 변화에 따라 optimal 수렴이 느려질 수 있다. 이를 방지하기 위해 Mean normalization 등과 같은 방법으로 x 값의 변화를 줄여준다.
ex) x1=size−10005000, x2=toilets−210
5. Polynomial Regression
복잡한 모델의 경우 일차함수로는 표현이 어려워진다. hθ(x)=θ0+θ1x1+θ2x2를 hθ(x)=θ0+θ1x1+θ2x1x2+θ3x21 등과 같이 차수를 늘려 사용하면 복잡한 모델을 표현할 수 있게 된다.
항상 차원을 높이는 것이 좋지만은 않다. 너무 높이게 될 경우, Overfitting 문제를 야기할 수 있다. 반대로 너무 낮을 경우, Underfitting 문제를 야기할 수 있다.
6. Normal equation
Gradient descent 말고 일반 행렬식을 이용해 풀 수 있는 방법도 있다.
XΘ=Y
XTXΘ=XTY
(XTX)−1XTXΘ=(XTX)−1XTY
Θ=(XTX)−1XTY
α처럼 huristic part가 없고 계산으로 바로 구할 수 있다는 점에서 Gradient descent보다 장점을 가지지만, 복잡한 행렬식을 계산해야 한다는 점과 n이 크면 속도가 매우 느리다는 점이 단점이다.
일반적으로 data가 크며 모델 생성 속도를 중시한다는 점에서 Gradient descent를 더 사용한다.
7. 필기

'Study > Machine Learning' 카테고리의 다른 글
6. KNN classifier (0) | 2023.04.20 |
---|---|
5. Neural Network (0) | 2023.04.20 |
4. Overfitting (2) | 2023.04.20 |
3. Classification (0) | 2023.04.20 |
1. Introduction (0) | 2023.04.20 |