Template Matching
Kernel을 통해서 pattern이 있는지 찾는 방법이다. Filter와 Image가 유사할수록 큰 값을 가진다.
c(x,y)=∑s∑tω(s,t)f(x+s,y+t)
Normalization할 수 있다.
c(x,y)=∑s∑t[ω(s,t)−ωmean][f(x+s,y+t)−fmean]∑s∑t[ω(s,t)−ωmean]2[f(x+s,y+t)−fmean]2
size와 rotation의 normalization은 복잡해서 다른 방법이 필요하다.
*2D rotation
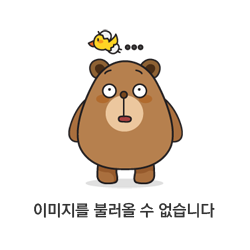
Linear Interpolation&Extrapolation
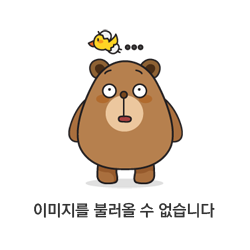
- Interpolation : 확대했을 때, hole이 생기는데 이 hole의 값을 predict할 때 사용한다. 여러 보간법이 존재한다.
- Extrapolation : 축소했을 때, 사용된다.
Fourier Descriptor
Object의 size와 rotation 파악할 때 쓰는 것으로, Pre-processing의 목적으로 주로 사용된다.
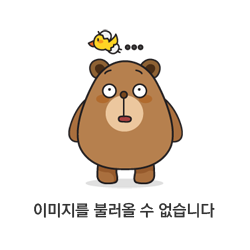
s(k)=x(k)+iy(k)
Fourier Transform...
a(u)=∑N−1k=0s(k)exp(−2πukN)
u는 object's curve의 frequency라고 보면 된다. 따라서 u가 작으면 approximated shape에 관한 정보이고 u가 크면 detailed shape를 표현한다. 구체적인 정보는 다음과 같다.
a(0) | position of center of gravity translation infromation |
a(1) | size of the shape alone = shape is circle, r = a(1) scaling information |
Phase | rotation of this perturbation show where the action is performed on a(1) circle |
Others | a(1) circle에 자잘한 변화(alteration)를 줌 |
Negative value | positive와 opposite effect |
Fourier descriptor로 할 수 있는 것은 Translation, Scaling, Rotation이며 모르는 것은 Object의 Position, Size, Orientation이다. 모르는 부분은 뒤에서 배우는 Homography를 통해서 알 수 있다.
Pre-processing
Translation : a(0)=0
Scaling : a′(u)=a(u)/a(1)
Rotation Invariance : a′(u)=a(u)/ejϕ
'Study > Computer Vision' 카테고리의 다른 글
9. Camera Calibration (2) | 2023.05.10 |
---|---|
8. Homogeneous Transformation Matrix (0) | 2023.05.10 |
6. Bayes Classifier (0) | 2023.05.10 |
5. Object Recognition (0) | 2023.05.10 |
4. Segmentation (0) | 2023.05.10 |